An interview with Jeremy Klein, Chair of RADMA and a technology and business consultant. Jeremy is track chair for ‘Contextual Specificity in R&D Management’ in the Innovation and Digital Transformation section of the R&D Management Conference 2022.
We caught up with Jeremy and asked him why ‘contextual specificity’ is important.
What do you mean by contextual specificity?
Yes, it is an unusual term but we got some interesting papers in the 2021 conference, so we decided to investigate this further in 2022.
It’s an odd thing that the academic discipline of R&D Management seems to gloss over the question of which technology we are trying to research or manage.
Practitioners know very well that managing pharmaceutical drug development is a world apart from managing software development. The timescales are different, the costs are different and the people are different too. Academics know this too, but it’s pretty low down in their priorities.
The basic idea is that sometimes, when we are looking at the management of technology or of R&D, we have to pay attention to the specific contexts involved – and most importantly, the specific technologies.
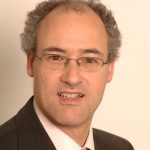
So you are saying that we need to look more closely at the fields of technology – like nano-technology or artificial intelligence?
Exactly. But even here, the two terms you’ve mentioned are actually very broad.
Take nano-technology. I was sitting in a conference a few years ago and there was a paper about business models for nano-technology. At the time I’d been doing some work in the field and I knew that nano-technology was not really a singular ‘technology’. Rather, it was a useful catch phrase for policy makers that rolled together all sorts of different nano-technologies: nano-motors, nano-actuators, nano-particles, nano-coatings and so on.
You see, there are many different sorts of nano- technology and to imagine that they should be addressed and understood as if they were one thing misses the point. In fact, it’s the variety and richness of technology that makes managing R&D so interesting.
What does this mean for the management of R&D?
The most important implication is that there’s a sort of interaction between technologies, contexts and management tools.
If we look at the history of, say, Agile software development we find that it arose in a very specific context. The old way of running software projects wasn’t delivering results within the timescales that the emerging internet businesses needed. But software, by its nature, could be managed through Agile methodologies.
So you have this close relationship between the technology, which is software, and a management technique, Agile. We see this in other places too. Like the invention of technology readiness levels for space systems at NASA.
But people are trying to extend the use of Agile out of software aren’t they?
True, but I’m not sure it’s settled yet. To some extent Agile is a fashion and we know that people sometimes take fashions too far. There was an article in the Harvard Business Review a few years back basically arguing that Agile could be used just about everywhere. But more recent academic work is suggesting that Agile has its place but sometimes it’s not going to fit.
Do you have any topics on your wish list of papers for the 2022 Conference?
There is one topic I’d love to see being explored. It’s the R&D implications of ‘digital twins’. To be honest, I’d never heard of digital twins till a couple of years ago but now they are cropping up everywhere. You get digital twins of machines, factories, ports and even people. They can be powered with artificial intelligence too.
So here is an emerging phenomenon but I’m not aware of any research in the managerial implications of digital twins.
Another big area of course is artificial intelligence. I think there is a lot that needs to be done on how AI will affect the process of product development. Traditional engineering approaches to product development involved creating prototypes and testing them. They either worked or they didn’t. AI makes the question of performance less clear. The same AI software may not work with one set of training data and work well with another. If you don’t know if a system works, then making decisions about it becomes more ambiguous. But AI also enables you to make predictions where you don’t have well-formulated models.
So AI is having some really fascinating impacts. I think AI may challenge the go/no go paradigm of our current ‘stage gate’ processes.