AI isn’t just a tool for faster research – it’s a catalyst for discovery. To support this, Elsevier, a global leader in advanced information and decision support, has launched ScienceDirect AI, a cutting-edge generative AI tool for researchers.
It is designed to transform the way researchers work by enabling them to instantly extract, summarize and compare trusted insights from millions of full-text articles and book chapters on ScienceDirect, the world’s largest platform for trusted, peer-reviewed research.
Stuart Whayman, President of Corporate Markets at Elsevier, explains the importance of the new tool.
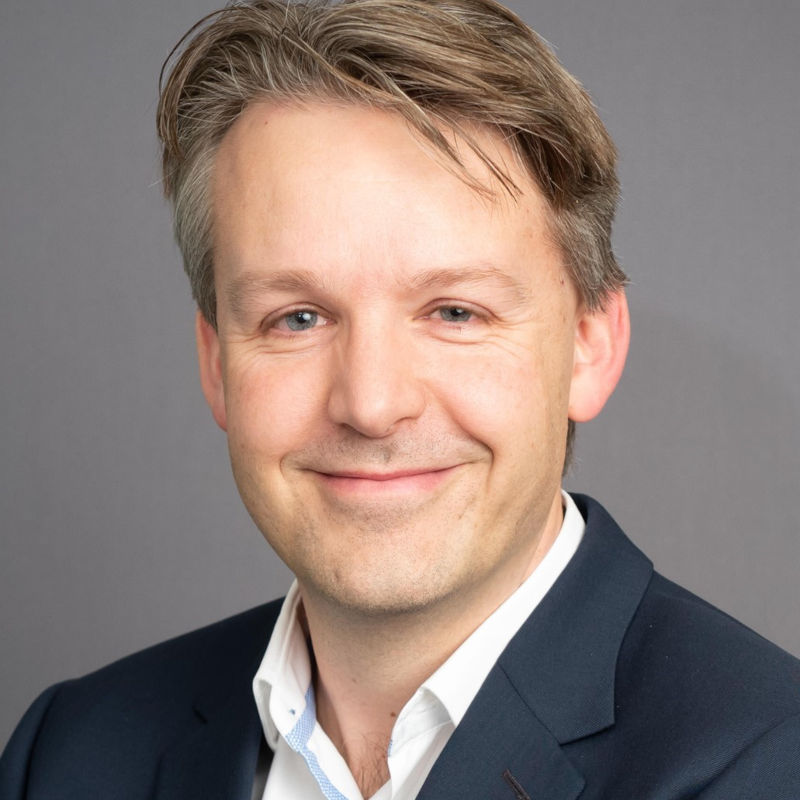
AI is transforming research-intensive industries, from pharmaceuticals and chemicals to aerospace and automotives, by accelerating product development and decision-making.
Researchers no longer want to rely solely on manual search and raw data. Instead, they expect to use natural language search via user-friendly platforms to query findings. Sophisticated AI-powered tools are delivering on this demand, making it easier to surface insights, compare findings, and generate new hypotheses in a fraction of the time, whether that’s for drug discovery, advanced materials, or next-generation vehicle design.
However, the rigorous standards of evidence-based and regulated industries mean AI-generated insights must meet high thresholds for accuracy and accountability. In fields where decisions have scientific, safety, or regulatory implications, every insight must be verifiable and reproducible.
Integrating AI into R&D workflows requires more than just speed, it demands robust validation, deep domain expertise, and strict adherence to industry standards to maintain research integrity and ensure compliance.
Four challenges of using AI in R&D
1: Hallucination and misinformation
AI’s ability to analyze and summarize vast datasets is powerful, but not flawless. Generative AI models can produce hallucinations, especially models that are publicly available and trained only on web data. Hallucinations are factually incorrect or misleading outputs that can derail projects and lead to misinformed decisions, regulatory issues, and wasted resources. Corporate researchers are acutely aware of this challenge, with 84% reporting concern that AI may cause critical errors or mishaps.
2: Lack of domain-specific knowledge
Many publicly available commercial AI tools are designed for broad applications and lack technical expertise in specialized industries. This can result in misinterpretations, especially when it comes to domain-specific scientific and technical language.
3: Traceability and reproducibility of AI-generated insights
R&D-intensive industries rely on evidence-based decision making. Verifiable and reproducible insights are essential, yet many AI platforms function as “black boxes” with limited transparency into how a model has arrived at its answer. As regulations like the EU AI Act introduce stricter requirements for accountability and risk management, ensuring AI outputs are traceable and evidence-based is increasingly critical.
4: Shadow AI use
As AI tools become more accessible, researchers and engineers may turn to unapproved AI models without proper oversight – a phenomenon known as ‘shadow AI’. Research finds more than half (55%) of corporate researchers are prohibited from uploading confidential information into public generative AI platforms, yet informal AI use persists. This risks data breaches, IP leaks, and regulatory non-compliance.
How to build a responsible AI platform for R&D
Instead of restricting AI, organizations must proactively embrace its use by adopting trusted, purpose-built tools with strict guardrails. This responsible approach allows businesses to reap the benefits of AI’s faster workflows and innovation capabilities, while maintaining compliance, protecting sensitive data, and mitigating risk.
For AI to be a valuable asset in R&D, there are several key attributes platforms must have. Firstly, a well-designed AI platform should be built for a specific industry, trained on trusted domain-specific data sources, and capable of accurately interpreting technical language. Achieving this requires input from subject matter experts, who can verify reliable data sources and ensure AI aligns with regulatory standards. Security and compliance must also be a priority; companies cannot risk leaking trade secrets, whether it’s a new drug formula or proprietary battery technology.
Usability is also important – companies should invest in AI platforms that excite researchers and enable them to analyze information in intuitive ways. One valuable feature is built-in comparative analysis, which enables the rapid summary of various methodologies and results across multiple papers. As the example in Figure 1 shows, a researcher in food science has used AI to streamline literature review by extracting key insights to significantly reduce the time spent manually analyzing research.
Another necessary function is the ability to engage conversationally with AI about data. Rather than passively consuming research, an AI-powered reading assistant encourages deeper exploration by suggesting follow-up questions based on the context of a study. For instance, when reviewing an article on brain tumor imaging, AI might prompt the researcher to ask: “How do these imaging advances contribute to understanding the prognosis of brain tumors?” This dynamic interaction ensures researchers don’t just find answers – they discover new lines of inquiry (fig.2).
Finally, to increase researchers’ and stakeholders’ trust in AI, generated insights should be fully referenced, linking back to peer-reviewed sources so researchers can verify claims and reproduce findings. Ideally, these references should direct users to the exact paragraph or line of text the AI has drawn from. Such audit trails will also help with compliance later down the line, as AI regulations continue to evolve.
Smarter AI, stronger research
The above examples demonstrate how successful integration of AI in R&D and product development hinges on a balance of technology, data, and domain expertise. Advanced AI models can process vast datasets quickly, but without trusted data sources and expert validation, organizations risk generating misleading or incomplete insights. By prioritizing scalability, trustworthiness, and compliance, businesses can fully harness AI’s potential while safeguarding technical and research integrity.
When deployed responsibly, AI isn’t just a tool for faster research – it’s a catalyst for discovery, unlocking novel insights that might otherwise remain buried in vast stores of information. Those who embrace AI with a strategic, evidence-driven approach will be best positioned to turn data into real-world innovation.